Artificial Intelligence (AI) and machine learning are transforming various sectors, driving numerous technological innovations. In this article, we will explore the fascinating realm of AI acronyms introduced by Alaikas. We will discuss the significance of these acronyms, their advantages, and the challenges they present.
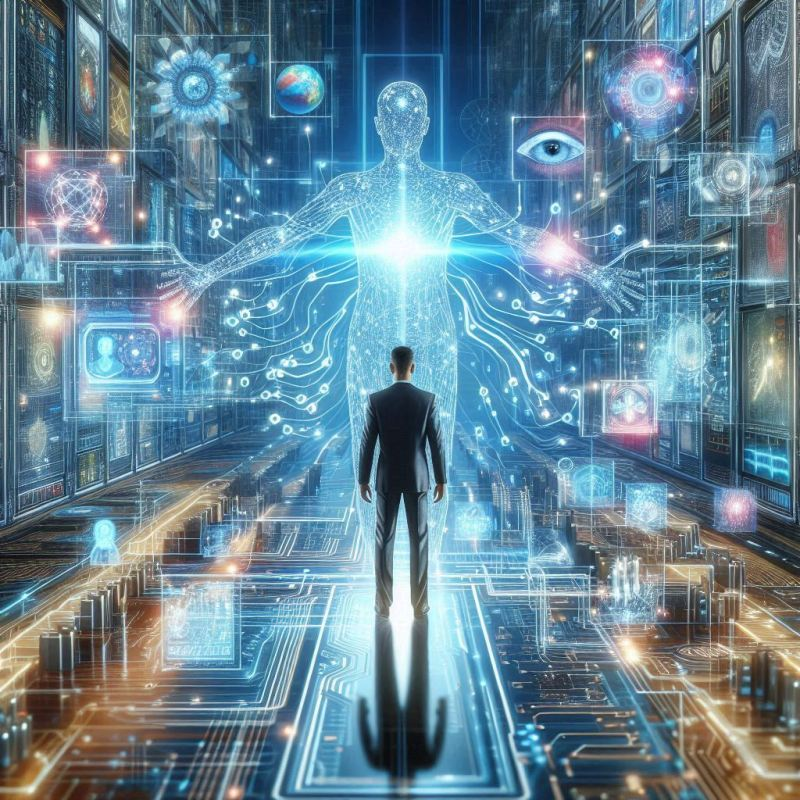
Recent Advancements In AI and Machine Learning
Advancements in Healthcare Artificial Intelligence (AI) and machine learning are making significant strides in healthcare. AI-driven algorithms are now capable of predicting medical conditions and tailoring treatments to individual needs. For instance, AI technologies are being employed to identify early indicators of diseases such as cancer, enabling prompt and effective medical interventions.
Transformations in the Financial Sector The financial industry is experiencing notable improvements thanks to AI. Machine learning algorithms enhance fraud detection by analyzing transaction patterns to pinpoint suspicious activities. Additionally, AI-powered chatbots are revolutionizing customer service by providing immediate support, streamlining banking operations, and boosting overall efficiency.
Innovations in Autonomous Vehicles The development of self-driving cars represents a groundbreaking achievement. Companies such as Tesla and Waymo are harnessing AI to create vehicles that operate autonomously, without the need for human drivers. This innovative technology has the potential to reduce traffic accidents and enhance the efficiency of transportation systems.
Basic Artificial Intelligence Acronyms
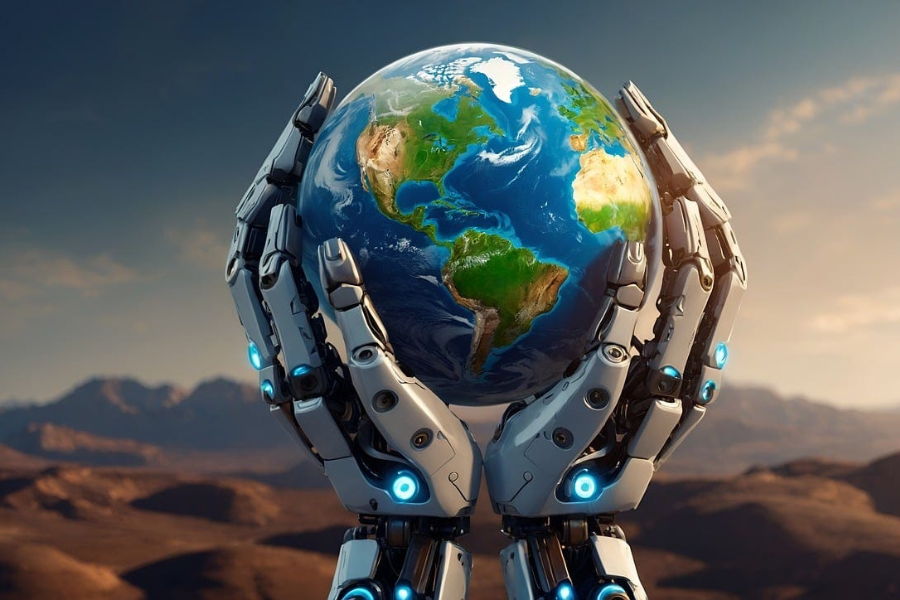
Understanding Key AI Concepts
Artificial Intelligence (AI) Artificial Intelligence encompasses the creation of machines that mimic human cognitive functions. These systems are designed to perform tasks such as speech recognition, decision-making, and language translation, emulating human-like thinking and problem-solving abilities.
Machine Learning (ML) Machine Learning, a subset of AI, focuses on developing algorithms that enable computers to learn from and make predictions based on data. Essentially, ML allows computers to improve their performance over time by analyzing data patterns, similar to how humans learn from experience.
Deep Learning (DL) Deep Learning is a specialized branch of Machine Learning that employs neural networks with multiple layers—hence the term “deep.” This approach is particularly effective for complex tasks such as image and speech recognition, where it can discern intricate patterns and features within large datasets.
AI Applications
Key Technologies in AI:
Automatic Speech Recognition (ASR) Automatic Speech Recognition (ASR) technology enables computers to understand and interpret human speech. This capability is crucial for applications such as virtual assistants, transcription services, and voice-controlled systems, allowing machines to process and respond to spoken language.
Text-to-Speech (TTS) Text-to-Speech (TTS) technology converts written text into spoken words. This allows computers to vocalize text, making it accessible to individuals with visual impairments or reading difficulties. TTS systems enhance accessibility by enabling the auditory reading of written content.
Optical Character Recognition (OCR) Optical Character Recognition (OCR) is a technology that transforms images of typed, handwritten, or printed text into machine-readable text. This process is essential for digitizing physical documents, allowing them to be edited, searched, and managed electronically.
The Most Common Artificial Intelligence Acronyms By Alaikas
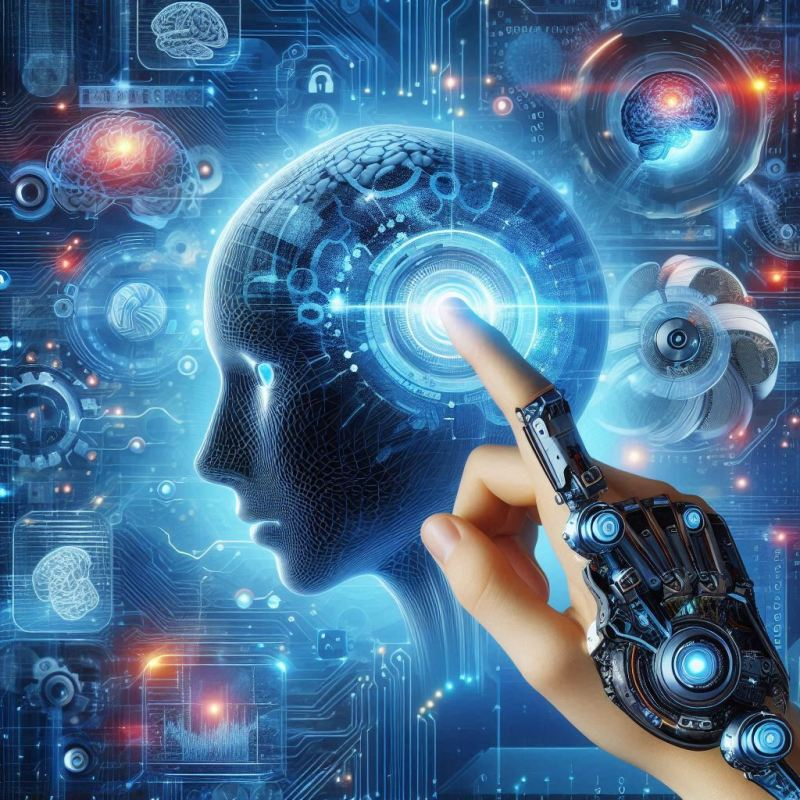
Artificial Intelligence (AI)
Artificial Intelligence (AI) is a broad field dedicated to creating machines that exhibit human-like cognitive functions. AI encompasses a range of capabilities including learning, reasoning, and problem-solving, aiming to mimic various aspects of human intelligence.
Machine Learning (ML)
Machine Learning (ML) is a subset of AI focused on developing algorithms that allow computers to learn from and make decisions based on data. Unlike traditional programming, ML enables systems to improve their performance over time through experience and data analysis.
Deep Learning (DL)
Deep Learning (DL) is a specialized area within machine learning that utilizes neural networks with multiple layers. This approach excels at identifying complex patterns in large datasets and is particularly effective in tasks such as image and speech recognition.
Natural Language Processing (NLP)
Natural Language Processing (NLP) is a branch of AI that deals with the interaction between computers and human languages. NLP techniques are employed for tasks such as text analysis, language translation, and speech recognition, enabling machines to understand and process human language.
Convolutional Neural Network (CNN)
Convolutional Neural Networks (CNNs) are a type of deep neural network specifically designed for processing and analyzing visual information. They are widely used in applications such as image and video analysis due to their ability to capture spatial hierarchies in visual data.
Recurrent Neural Network (RNN)
Recurrent Neural Networks (RNNs) are designed to handle sequential data and are particularly useful for tasks that involve time series analysis or natural language processing. RNNs can maintain information over time, making them ideal for tasks where context and order are important.
Artificial General Intelligence (AGI)
Artificial General Intelligence (AGI) refers to a theoretical form of AI that possesses the ability to understand, learn, and apply intelligence across a broad range of tasks, similar to human cognitive abilities. AGI represents a future goal for AI research, aiming for a level of versatility comparable to human intelligence.
Support Vector Machine (SVM)
Support Vector Machines (SVMs) are supervised learning models used for classification and regression tasks. They work by finding the optimal hyperplane that separates different classes in the data, making them effective for tasks involving pattern recognition and data classification.
Generative Adversarial Network (GAN)
Generative Adversarial Networks (GANs) consist of two neural networks—a generator and a discriminator—that work in tandem to create realistic synthetic data. The generator produces new data samples, while the discriminator evaluates their authenticity, leading to the generation of highly realistic data.
Application Programming Interface (API)
Application Programming Interfaces (APIs) play a crucial role in AI by enabling different software systems to interact with AI models and services. APIs facilitate integration and functionality, allowing developers to incorporate AI capabilities into their applications seamlessly.
Advanced AI Acronyms By Alaikas
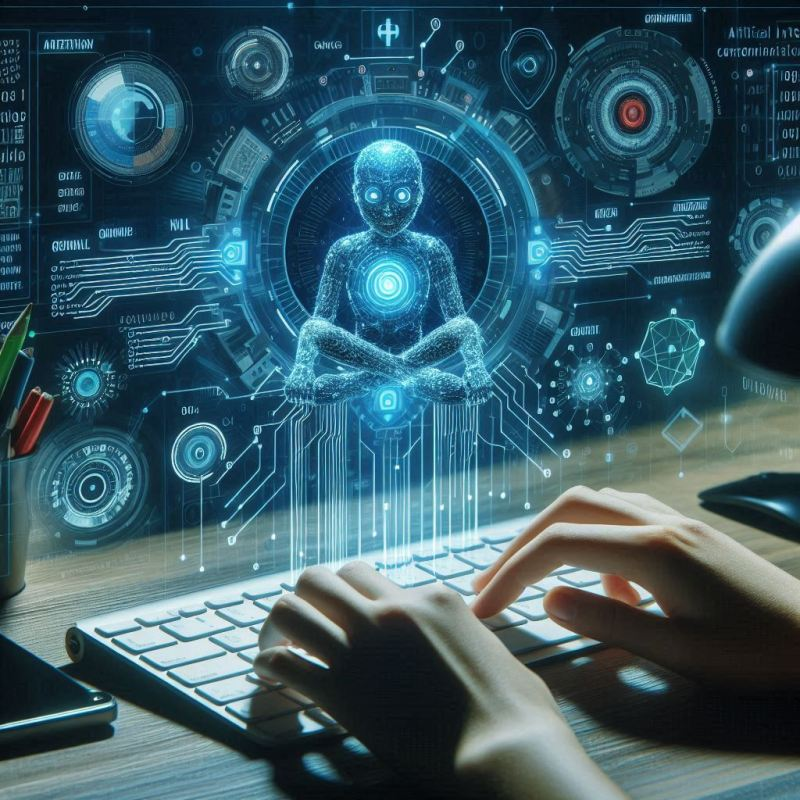
BERT: Bidirectional Encoder Representations from Transformers BERT, which stands for Bidirectional Encoder Representations from Transformers, is a deep learning model created by Google for processing natural language. This model enhances the understanding of context in text by considering the bidirectional relationships between words, significantly improving language comprehension and text analysis.
GPT: Generative Pre-trained Transformer Generative Pre-trained Transformer (GPT) is a highly advanced text generation model developed by OpenAI. GPT excels in generating human-like text and is pre-trained on vast amounts of data. This model can be fine-tuned for specific applications such as text completion and creative writing, demonstrating its versatility in natural language processing.
RL: Reinforcement Learning Reinforcement Learning (RL) is a dynamic AI approach where an agent learns to make decisions by receiving rewards or penalties based on its actions. This learning technique is used in various fields, including robotics and game development, where the agent continuously improves its performance through trial and error.
TTS: Text-to-Speech Text-to-Speech (TTS) technology converts written text into spoken words. This tool is commonly used in virtual assistants and accessibility applications, such as screen readers for visually impaired users, to facilitate interaction and improve accessibility by providing spoken output for written content.
Frequently Asked Questions (FAQs)
1. What is BERT and how is it used?
BERT, or Bidirectional Encoder Representations from Transformers, is a natural language processing model developed by Google. It enhances text understanding by analyzing the context of words in both directions (left-to-right and right-to-left). BERT is widely used in tasks like question answering and language inference to improve the accuracy of machine understanding.
2. How does GPT differ from other text generation models?
Generative Pre-trained Transformer (GPT) is notable for its ability to produce coherent and contextually relevant text. Unlike some other models, GPT is pre-trained on a vast dataset and can generate human-like text based on prompts. Its versatility allows it to be used in a variety of applications, from creative writing to automated content generation.
3. What is reinforcement learning and its primary applications?
Reinforcement Learning (RL) involves training an agent to make decisions by rewarding desirable actions and penalizing undesirable ones. This method is commonly used in areas like robotics, where the agent learns to perform tasks through repeated trials, and in gaming, where it helps develop strategies and improve performance.
4. How does Text-to-Speech (TTS) technology benefit users?
Text-to-Speech (TTS) technology converts written text into spoken audio, which benefits users by providing an auditory option for reading text. This is particularly useful for individuals with visual impairments or reading difficulties, as it enables them to access written content through sound.
5. Can BERT and GPT be used together in a project?
Yes, BERT and GPT can complement each other in projects. For example, BERT can be used for understanding and interpreting context, while GPT can generate or complete text based on that understanding. Integrating both models can enhance overall performance in applications requiring complex language processing and generation.
Conclusion
Artificial Intelligence (AI) and its various acronyms, such as BERT, GPT, RL, and TTS, represent significant advancements in technology that are shaping the future of numerous industries. Each acronym stands for a specialized aspect of AI, contributing to the broader field of machine learning and natural language processing.
Understanding these acronyms helps in grasping the capabilities and applications of modern AI technologies. From enhancing text comprehension with BERT to generating human-like text with GPT, and from decision-making in robotics through RL to converting text to speech with TTS, these innovations are driving progress and creating new possibilities across different domains.
As AI continues to evolve, staying informed about these technologies and their functionalities will be crucial for leveraging their full potential and addressing emerging challenges in the field.